Large language models (LLMs) are a hot topic at the moment, which is hardly surprising given how quickly ChatGPT, Claude, and many others have captured the public's attention.
But even before the current LLM hype cycle was in full swing, our team was experimenting and trying to unearth the technology's potential.
It means we’ve been working with LLMs in our digital humans for many years: building tools like Synapse to help make conversations more brand-safe and relevant, and Synanim to make conversations more accessible and fun.
So we thought we'd ask some of our in-house experts to look into their crystal balls and predict what's next for LLMs in 2025 and beyond.
Here's how they think these generative AI models will evolve:
LLMs must move beyond the math to be creative
Tyler Merritt, Field CTO
LLMs are still largely constrained to the logical sector. That means we try to solve everything with math and numbers. But there's still a lot we don't understand about the human brain, and it's clear to me that math is not the right language to describe feelings like nostalgia or the creative process.
Creativity happens when we mix experience with new insights, inspiring us to think about things in a way we've never done before. So language models are inevitably going to struggle if they're simply using math to describe things that we already know. How will we come up with a model that can replicate creativity?
Perhaps an injection of randomness is what's needed. Start throwing models curveballs and see what the results are. For example, what happens if we feed random, dissociated content into models at various points – will they start to behave as if they're having ideas of their own? Will the models feel more creative?
Let's start trolling the machine, so to speak. And in doing so, we might accidentally chance on something that starts to look and feel more like a human.
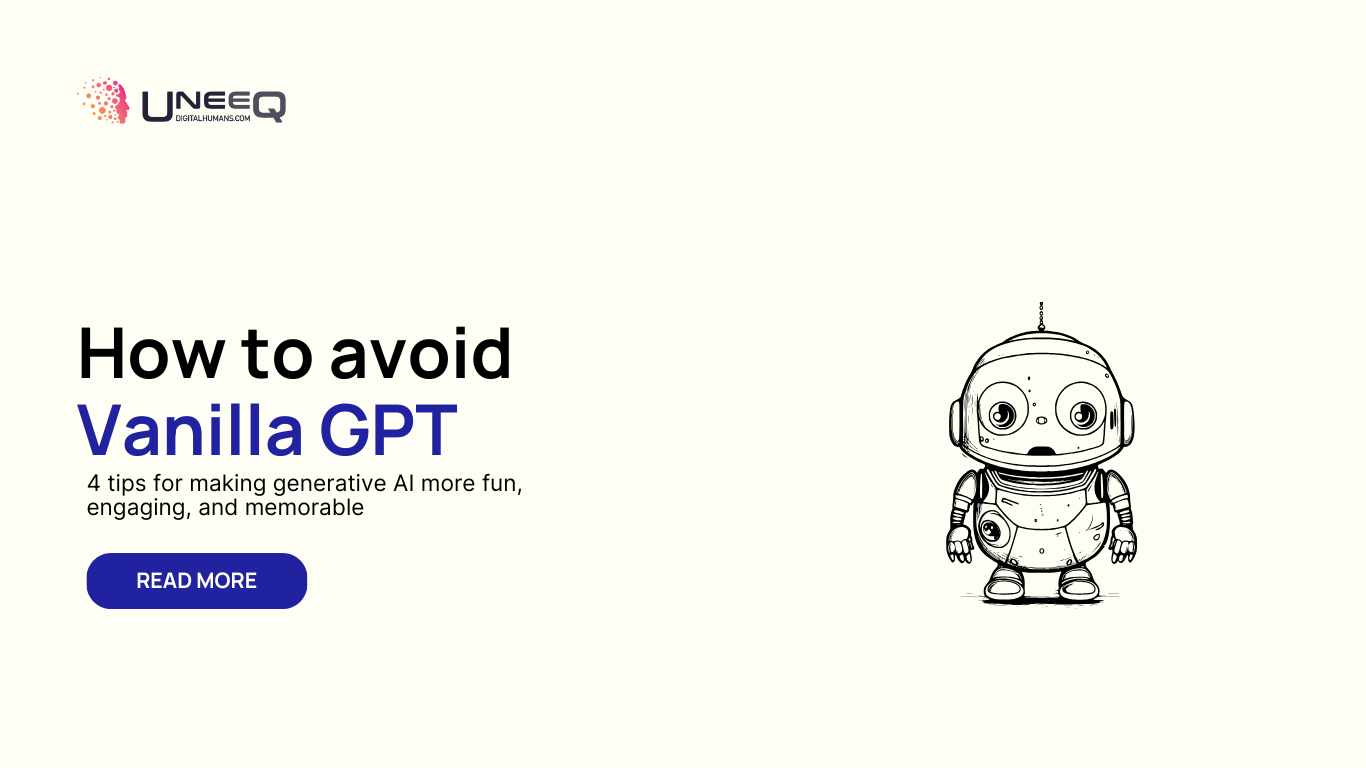
There will be no One Model to rule them all
Jason Catchpole, Head of AI
LLMs are likely to come in a variety of shapes and sizes over the next few years.
We'll see both extremes of the spectrum – smaller models that run in more places, such as on smartphones, as well as massive models that only the biggest companies have the resources to train. That means we'll have different models focused on different things, rather than One Model to rule them all.
I suspect there will also be much more training data from outside English-speaking/Western countries, which will help models overcome certain types of bias. The open sourcing of models will continue, however, with smaller businesses and start-ups likely building on the foundation models of other companies.
Over the long term, there will need to be new, more data- and energy-efficient approaches to LLMs in particular and AI systems in general as they become larger and more sophisticated. On the flip side, this should lower the requirements for the amount of data needed, opening the door for smaller companies to train models more easily.
AI is cyclical, but LLMs are here to stay
Paul Haggo, Platform Team Lead
For over 60 years, there have been waves of excitement about what AI can achieve. It's common to see an early period of hype, followed by disappointment when the technology doesn't quite match the initial hype. Eventually, funding and R&D begins flowing again, and the cycle starts over.
This is likely to keep happening. However, LLMs have managed to get over a tricky hump that few AI technologies have previously managed – they have clear utility. The fact that ChatGPT has become a household name in such a short time is ample evidence of this. In other words, I think LLMs are here to stay.
That doesn't mean they won't face the same trough of disillusionment as other hyped technologies. There are lofty expectations for LLMs, and it's going to be difficult to meet all of them. Still, we are already seeing companies (like UneeQ) that are leveraging this tech for real-world utility.
As for functionality, I expect audio and video to become the main way that people interact with LLMs in the future. A good example would be using your phone's video to stream to an AI model that gives you visual and verbal instructions on how to assemble furniture or repair products.
LLMs will have a face, voice, and personality
Ashley Johnson, Senior Director of Marketing
Like Paul, I think the way that people interact with LLMs will change. Very soon it'll be much more voice based. That's just how most people prefer to interact, especially when it comes to long-form content.
Humans crave authentic interactions and conversations. I've also noticed how Siri now sometimes says 'You're welcome' when I thank her.
People want to be able to pick up their phone and have a genuine voice-based conversation on a topic. Using Siri as an example again, I recently asked her how you know when a cantaloupe is ready to be taken off the vine. It ended up being a frustrating experience!
She replied with information about how to know when it's ripe in the store, rather than when gardening. So I ended up having to take my garden gloves off and use Google and YouTube to find the information I needed. I'd much rather have a friendly voice that a) knew what I was talking about and b) could chat me through it while I'm out in my garden.
That's why I expect LLMs like ChatGPT and Llama to start having unique voices and names soon, making them more personified. After that, we'll begin to see LLMs with faces, personalities and their own mannerisms, much like digital humans.

Better knowledge injection will become crucial
Gus Matheson, Director of Technical Solutions
There have been many great talking points from everyone so far, much of which I agree with! Another angle to explore is what technical changes will be needed as we approach the ceiling of LLM capabilities.
Platforms like ChatGPT are prone to 'hallucinations', which means they produce false information if they're struggling to answer questions that go beyond their training data. So, knowledge injection techniques, such as Retrieval-Augmented Generation (RAG), are used to improve the accuracy and reliability of LLMs.
This enables them to retrieve external information in real-time to give better, more specific answers. However, true knowledge injection at run-time is increasingly crucial as LLMs grow. As a result, traditional techniques will quickly become outdated as their limitations are discovered.
Ultimately, I predict the way we control LLMs will become more important to creating a successful solution than developing more advanced training models – especially as we reach their limits.
We’ll have a term for generative AI search
Mark Hattersley, Senior Content Manager
I use ChatGPT every day to answer questions that I don’t think Google could answer quicker. Just this week I wanted to know how much water to use in a risotto – something I would have dreaded asking Google in the past, being forced to scroll through someone’s life story and the history of arborio rice just to get to the answer.
I’m certainly not alone in moving so much of my day-to-day question-asking away from Google and towards generative AI. But it’s too easy a prediction to say more people will start doing the same in 2025.
So instead, I predict there’ll soon be a new verb for performing a generative AI search – as you might say “let’s Uber” as opposed to “let’s take a ride-sharing service.”
At the moment, nothing has stuck in the public lexicon. “I’m going to ChatGPT it”. That’s a bit of a mouthful.
“I’m going to LLM it.” No chance.
Perhaps Google itself will launch such an impressive search product that we continue saying “I’m googling it”.
My guess is, at some point, the marketers at one of the major LLM companies will take charge and create a verb that’s so irresistible we’ll all start saying it. And those marketers will deserve a substantial pay rise.
Oh, and it’s one cup of rice to every three cups of water, if anyone was wondering.
LLMs will help create better digital characters
Victor Yuen, Senior Technical Animator
LLMs are incredible at gathering massive swathes of unstructured information and delivering it in ways that are useful to us. And as we get more familiar with LLMs, we'll be able to better apply them.
We've gone through a phase of trying LLMs with so many things, and I believe we'll see some incredible applications of the technology that provide us with huge utility. We already have Copilot for coding, and there's the potential for other interesting things such as having a personal expert cooking tutor or characters in games that are unscripted and emergent in their behavior.
Within my specific domain of animation and behavior, we want to see how we can manifest personality out of tools like LLMs. We're interested in simulating a dynamic and nuanced personality, and then generating behavior from this.
In order to react to stimulus, technologies need to understand or perceive things. Multi-modal LLMs are interesting in this area because they're showing pretty good capabilities at classifying and interpreting what's happening within images.
These capabilities are opening up an opportunity for us to create digital characters – including our own – that can operate in truly immersive environments.
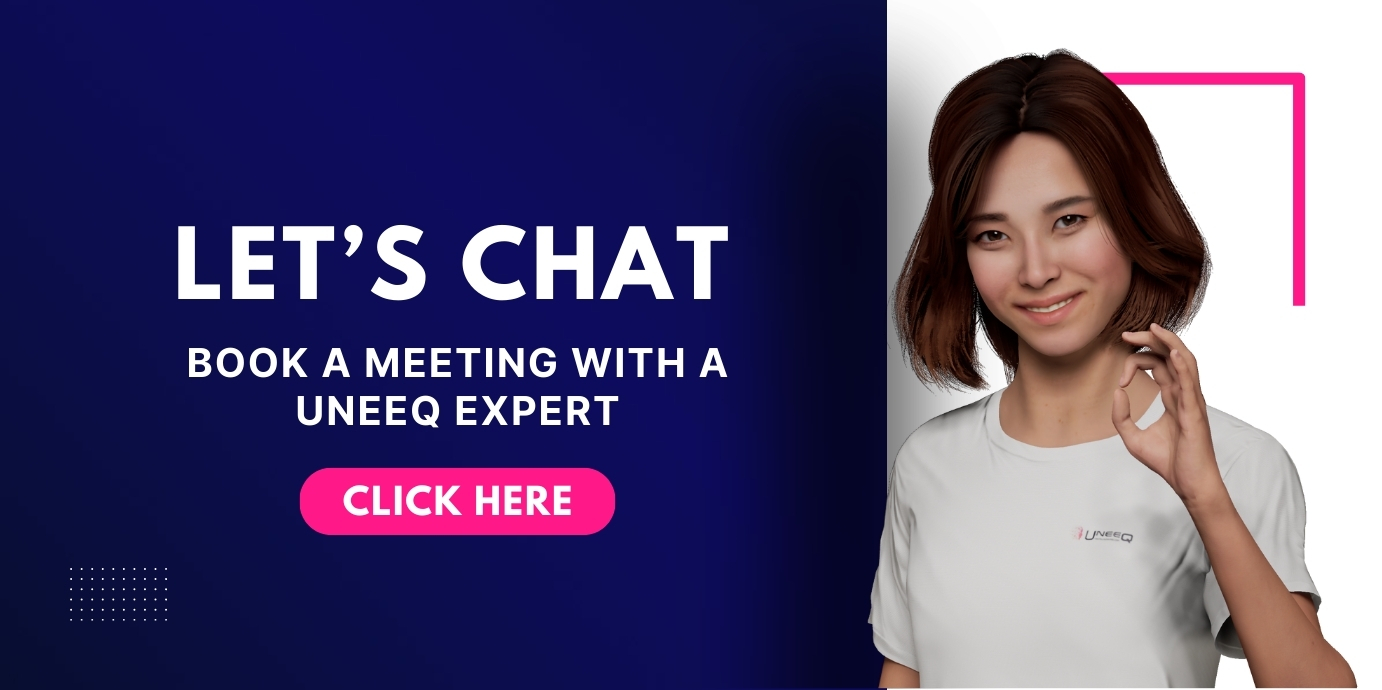